The adoption of artificial intelligence (AI) among Estonian private sector companies is still in its early stages. According to the 2023 Digital Economy and Society Index (DESI), only 5.2 percent of Estonian companies had implemented AI, placing us below the European average. However, data from Statistics Estonia shows that in large enterprises, AI adoption already exceeds one quarter. Estonia has set an ambitious goal of reaching a 75 percent AI adoption rate by 2030.
The OECD defines artificial intelligence as a machine-based system that can generate predictions, recommendations, or decisions based on input data, thereby influencing physical or virtual environments. Unlike basic automation, which follows pre-set rules, AI systems are capable of learning and adapting to new situations.
AI can be categorized into “narrow” or task-specific AI – a relatively mature technology designed to solve specific problems – and “general” or generative AI, which has only emerged in recent years and is based on large language models. Narrow AI tools are already capable of processing large data sets to detect patterns and correlations, cluster information, and identify trends. These tools should be part of every modern organization’s standard toolkit. Recently, the emergence of generative AI – with ChatGPT being perhaps the most well-known example – has opened up entirely new possibilities. This is a field whose full potential we are only beginning to understand, whose implementation requires additional investments, and whose benefits could be truly revolutionary.
Even the tech giants developing large language models like GPT-4, Gemini, and LLaMa struggle to fully explain the capabilities of these systems. Their creators often don’t completely understand them either. Each day brings new insights and surprises regarding what these models can do. For example, researchers at OpenAI compare large AI models to living organisms – they run experiments and try to interpret the outcomes afterward. These models can generalize, perform calculations, and even learn new languages, but we are still far from fully grasping how they achieve these feats. Meanwhile, a flood of new software solutions based on these models is hitting the market daily.
The rapid development of generative AI opens the door to unprecedented opportunities that every forward-looking organization should seek to harness – whether by using commercially available services or building their own solutions on top of existing models. For instance, financial media company Bloomberg has developed an in-house generative AI model specifically tailored to the needs of the financial sector. Trained on both Bloomberg’s proprietary data and public datasets, BloombergGPT is capable of performing sentiment analysis, financial report analysis, and market forecasting – and it does so more effectively than general-purpose models.
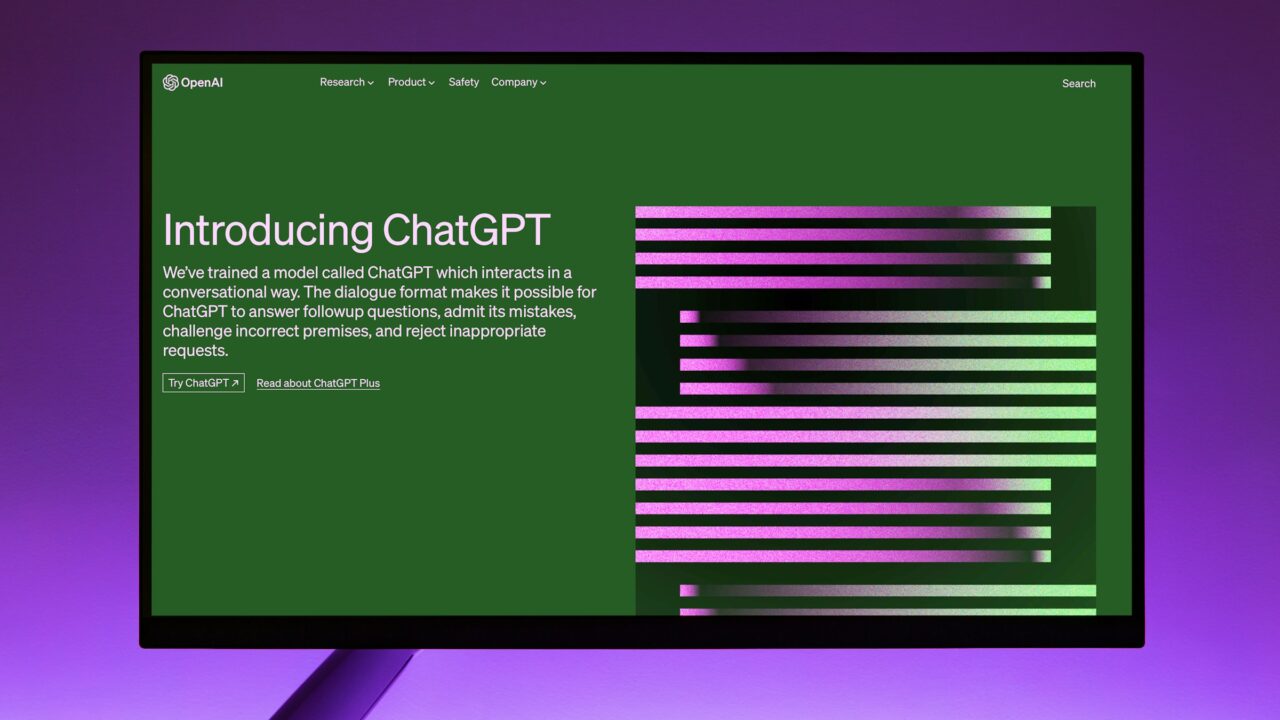
Even the tech giants developing AI struggle to clearly explain the capabilities of large language models | Photo: Andrew Neel/Unsplash
AI application areas in financial management
CFOs play an increasingly important role in companies’ strategic decisions, making them natural leaders and role models in the implementation of AI. In addition to core business processes, AI offers numerous opportunities to elevate financial management to a new level.
The financial world has already observed AI’s capability to deliver more for less (compared to humans). In a recent CitizenBank survey, one-third of U.S. mid-sized companies (comparable to large companies in Estonia) reported using AI for cash flow forecasting and financial analysis. New tools based on large language models further expand the potential for creating added value through AI – whether by developing future-proof strategic scenarios, creating and interpreting personalized reports, or rapidly adapting to novel fraud schemes.
In the context of financial management, experts most often highlight the following areas of AI application:
- Data analysis:
AI identifies patterns and trends in financial data, enabling faster and more accurate decision-making. Today, companies can already analyze vast amounts of data in real time using AI, including numerous variables that were previously deemed insignificant or too complex to use. Language models further allow the inclusion of non-traditional background data in analysis, enhancing the precision of assessments such as client creditworthiness and revenue potential. - Forecasting and scenario planning:
AI algorithms enable more accurate and automated forecasting by analyzing historical data, detecting patterns, and considering multiple influencing factors. With AI, it’s possible to generate an endless number of future scenarios to support strategic decision-making. Predictive models improve over time through machine learning, becoming more nuanced and better adapted to new trends, market shifts, and economic indicators. As a result, finance professionals receive forecasts that are not only more accurate but also responsive to a constantly evolving business environment. Generative AI adds the ability to incorporate significantly more varied background data into forecasts and interpret them for different user groups.
Research firm Gartner predicts that by 2028, forecasts will be continuous and autonomous in half of all large international enterprises thanks to AI. A practical example is Daimler, where an AI-based engine is already used to forecast KPIs (key performance indicators). This engine consists of a large number of algorithms, from which the system autonomously selects. Each time new monthly data becomes available, the AI generates millions of KPI forecasts, automatically validates them, and compares them with previous results. Based on this, the model selects the best algorithm or combination of algorithms for each KPI. To ensure continuous calibration, the system repeats this process every month and whenever the company introduces a new KPI.
- Reporting:
AI automates the generation of complex reports, reducing manual work and improving reporting accuracy. It also enables the creation of personalized reports and analytics in real time to serve various stakeholders. Some even believe that traditional reporting tools like Power BI will eventually disappear, replaced by conversational interfaces powered by generative AI – allowing users to ask questions and have the AI intuitively extract and present the data in the appropriate format.
Based on interactions with Estonian companies, it can be said that AI is currently applied in financial management in narrow areas, such as predicting customer payment behavior, debt collection, or inventory levels. Advanced analytics and forecasting models are still used relatively rarely. There are also experiments underway to integrate language model-based chatbots into various stages of financial workflows.
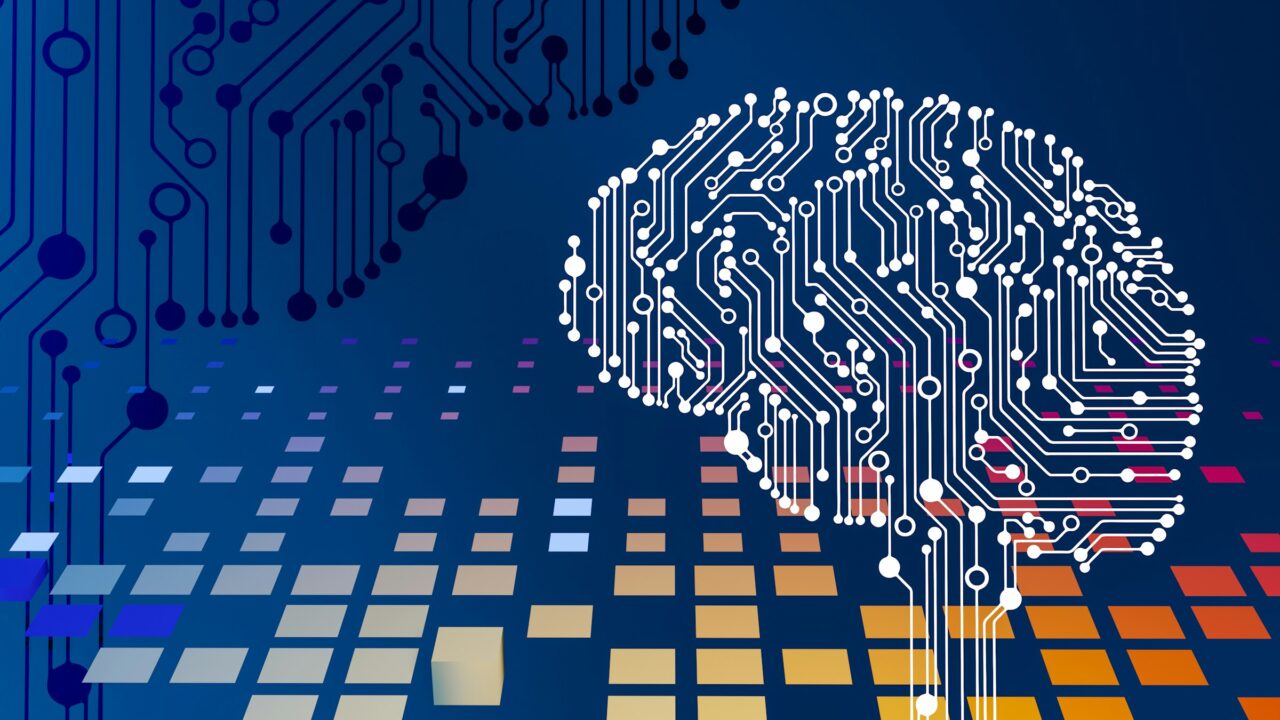
Based on interactions with Estonian companies, AI is currently being applied only in narrow areas of financial management | Photo: Steve Johnson/Unsplash
Successful AI implementation
According to a U.S. study by the consulting firm Deloitte, three key characteristics distinguish the frontrunners in AI implementation from the rest:
- Strategic planning:
Successful companies have a company-wide AI implementation plan in place. - Performance measurement:
Leading companies track the impact of their AI projects on revenue and customer experience, while also seeking new ways to apply AI. - Technological solutions:
Most companies begin their AI journey with existing software that already includes AI features. However, the frontrunners take a more diversified approach, leveraging AI-as-a-service and automated machine learning.
Next steps for adopting AI
If you’re thinking about how to accelerate your AI adoption, successful implementation requires decisive steps for driving major change:
- Clear responsibility:
Whether a company is large or small, achieving significant goals depends on accountability. A designated owner or team must be appointed to lead AI implementation efforts. - Building critical mass:
It’s essential that a sufficient number of employees understand AI principles and applications. - Training and awareness:
CFOs should ensure a regular training and awareness program on AI innovations and applications—for both themselves and their teams.
The arrival of large language models significantly amplifies the benefits of technology. Many fields, including financial management, are undergoing major transformations. But to succeed, conscious planning, investment, performance measurement, and continuous innovation are essential. The pace of change is rapid, and responses must be swift—yet deliberate.